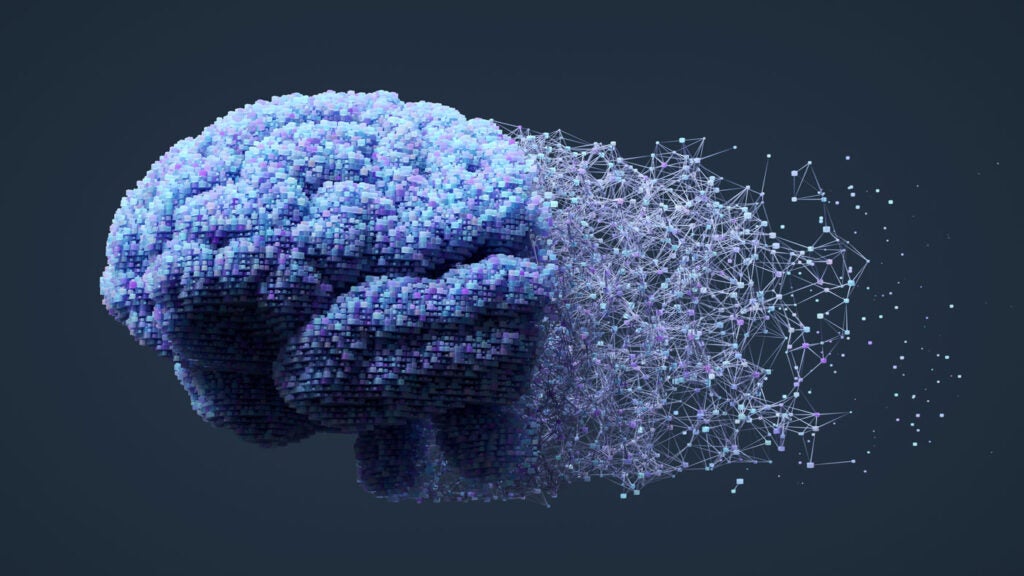
Researchers affiliated with Georgetown’s Center for Neuroengineering (CNE) have obtained a grant from the US Army Research Office to use advanced neuroimaging to test a computational model of how the brain builds on earlier learning to make the learning of new concepts easier. The resulting insights are not just expected to lead to improved learning algorithms for artificial intelligence but also provide insight into the brain mechanisms affected in semantic deficits in disorders such as dementia.
The new project is a collaboration between Maximilian Riesenhuber, PhD, professor of neuroscience at Georgetown University Medical Center and co-Director of the CNE, and Peter Bandettini, PhD, Chief of the Section on Functional Imaging Methods and Director of the Functional MRI Facility at the National Institute of Mental Health, combining the expertise of Riesenhuber’s group in computational modeling and electroencephalography (EEG) and Bandettini’s expertise in high-resolution functional magnetic resonance imaging (fMRI).
Humans can learn new concepts by noticing similarities to concepts they have already learned. As an example, imagine trying to convey the appearance of a platypus. One can provide a lengthy description of a platypus’s various features, such as describing fur, a bill, eyes, four legs, webbed feet, a tail and so on. Or one could more succinctly point out that a platypus looks like a cross between a duck, a sea otter, and a beaver.
“The brain is still unparalleled in its ability to build on prior learning to make it easier to learn new information, which is something computers are still struggling with,” says Riesenhuber. “Our experiments test predictions from a neuromorphic, that is brain-like, learning algorithm about what information is exchanged between different brain areas as people are learning new tasks, and how this new learning can make use of prior learning without interfering with it.”
The project will also make use of advanced neuroimaging techniques developed in Bandettini’s lab that make it possible to study neural processing at the fine spatial scale required to uncover the brain’s learning algorithms. “We have been developing techniques to use fMRI to measure neural activity in different cortical layers, and these techniques will enable us to probe what information enters a brain area, how it is transformed, and what is output to other areas,” says Bandettini.
A key feature of the project is that it uses more realistic objects than prior studies. “Objects in the real world are multimodal – that is, they have characteristic appearances in several different sensory modalities,” Riesenhuber adds. “For instance, dogs are furry, they bark, and sometimes, well, they smell. While most prior learning studies have used stimuli from a single sensory modality, our use of multimodal stimuli will enable us to better understand how the brain integrates different sources of information.”
“I’m excited that this award will enable leading researchers, Prof. Riesenhuber at GUMC and Dr. Bandettini at NIMH, to tackle one of the outstanding problems in brain inspired AI development – how does the brain incrementally learn new multimodal concepts without catastrophic interference? The challenge is inherently multidisciplinary, requiring both PIs’ unique expertise in high resolution neuroimaging, neurocomputation, and machine learning theory and implementation,” says Chou Hung, PhD, Program Manager of the Neurophysiology of Cognition Program at the Army Research Laboratory. “I expect that this foundational research will discover generalizable principles and theories that are necessary to develop and strengthen AI capabilities for the future Army.”